The Importance of Machine Learning Labeling Tools in Modern Business
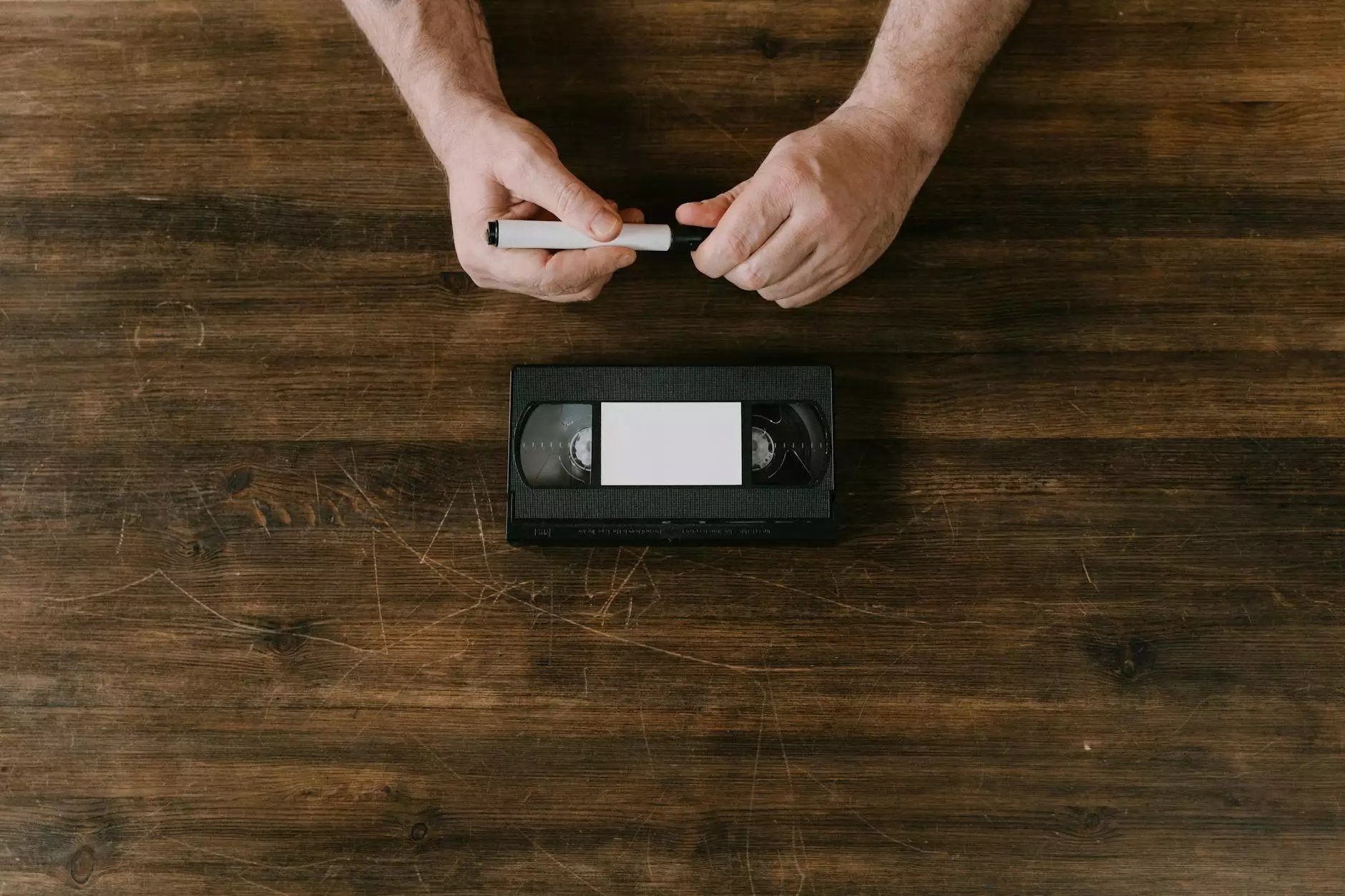
In today’s technology-driven landscape, businesses across all sectors are increasingly relying on artificial intelligence (AI) and machine learning (ML) to drive their operations. One integral component of this technology is the machine learning labeling tool. These tools play a vital role in enhancing the performance of machine learning models by ensuring that the data fed into these models is accurately annotated and labeled. This article explores the significance of these tools, the advantages they provide, and how businesses can effectively integrate them into their data annotation strategies.
Understanding Machine Learning Labeling Tools
A machine learning labeling tool is designed to assist in the annotation of data so that it can be utilized by machine learning algorithms. Data annotation is essential for supervised learning, where models learn from labeled input. These tools simplify the annotation process, making it faster and more efficient. They can handle various types of data, including images, text, audio, and video, which are critical in training machine learning models.
Why Data Annotation Matters
Before delving into the specifics of machine learning labeling tools, it's essential to understand why data annotation is a critical aspect of machine learning:
- Accuracy: Well-annotated data leads to models that perform better and make more accurate predictions.
- Training Efficiency: High-quality training data shortens the time required for model training, enabling faster deployment.
- Scalability: Automated labeling tools enable businesses to scale their data annotation processes efficiently.
- Consistency: Consistent labeling enhances the reliability of machine learning outputs, creating more robust models.
The Role of Machine Learning Labeling Tools
Machine learning labeling tools provide several functionalities that aid businesses in managing and executing data annotation projects. Here are some key roles they play:
1. Automation of the Annotation Process
Manual labeling can be time-consuming and prone to errors. Machine learning labeling tools use algorithms to automate aspects of the annotation process, allowing businesses to save time and reduce human error.
2. Collaboration Features
Many modern labeling tools include collaborative features that allow teams to work together seamlessly, which is essential for large-scale projects or distributed teams. These features include:
- Real-time collaboration: Teams can annotate and review data simultaneously.
- Version control: Track changes and manage different versions of annotation projects easily.
- Task assignment: Assign specific labeling tasks to team members, ensuring accountability.
3. Quality Control Mechanisms
To ensure the annotations are accurate, machine learning labeling tools often incorporate quality control mechanisms such as:
- Automated checks: Systems can automatically verify certain types of annotations.
- Reviewer roles: Designated reviewers can oversee the work done by annotators, improving overall quality.
- Feedback loops: Incorporate feedback to refine labeling guidelines continuously.
4. Support for Various Data Types
Different machine learning projects require the annotation of various data types. A versatile machine learning labeling tool supports:
- Image and video annotation: For computer vision tasks.
- Text annotation: For natural language processing.
- Audio annotation: For speech recognition applications.
Advantages of Using Machine Learning Labeling Tools
The adoption of machine learning labeling tools offers several advantages to businesses, making them indispensable in the realm of data annotation:
1. Increased Efficiency
By automating repetitive tasks and providing straightforward interfaces, these tools significantly enhance the efficiency of the annotation process. Businesses can annotate large volumes of data in a fraction of the time it would take manually.
2. Cost-Effectiveness
Reducing labor costs while increasing output is a primary goal for most businesses. Machine learning labeling tools streamline the annotation process, leading to lower operational costs over time.
3. Enhanced Accessibility
These tools often feature user-friendly interfaces, making them accessible to annotators who may not have technical expertise. This accessibility broadens the pool of potential contributors to machine learning projects.
4. Scalability
As the data needs of a business grow, machine learning labeling tools provide the scalability that is required to manage increasing data volumes without compromising on quality.
Best Practices for Integrating Machine Learning Labeling Tools
To gain the maximum advantage from machine learning labeling tools, businesses should consider the following best practices:
1. Define Clear Annotation Guidelines
Clear and concise guidelines ensure that all annotators understand how to label data accurately. This prevents inconsistency and enhances the quality of the annotated dataset.
2. Provide Training for Annotators
Investing time in training annotators on using the machine learning labeling tool effectively will optimize the annotation process and foster high-quality outcomes.
3. Implement Review Processes
Establishing a regular review process can catch errors early in the annotation workflow, leading to better overall quality and improved model performance.
4. Leverage Advanced Features
Take full advantage of the technology by using benefits such as automated labeling suggestions, AI-powered enhancements, and data analysis features that provide insights into the data annotation process.
Conclusion
As the demand for high-quality annotated data grows alongside advancements in AI and machine learning, investing in a robust machine learning labeling tool becomes crucial for businesses aiming to stay competitive. These tools not only enhance the data annotation process but also facilitate the development of more accurate and efficient machine learning models. By following best practices for implementation, businesses can fully leverage these tools, ensuring they remain at the cutting edge of technology and innovation.
About KeyLabs.ai
At KeyLabs.ai, we are committed to providing businesses with top-tier data annotation solutions through our innovative machine learning labeling tool. Our platform is designed to facilitate the smooth and efficient annotation of diverse data types, empowering organizations to harness the full potential of artificial intelligence. Explore our services to elevate your data strategies and achieve remarkable outcomes.