Enhancing Business Efficiencies with Machine Learning Data Labeling
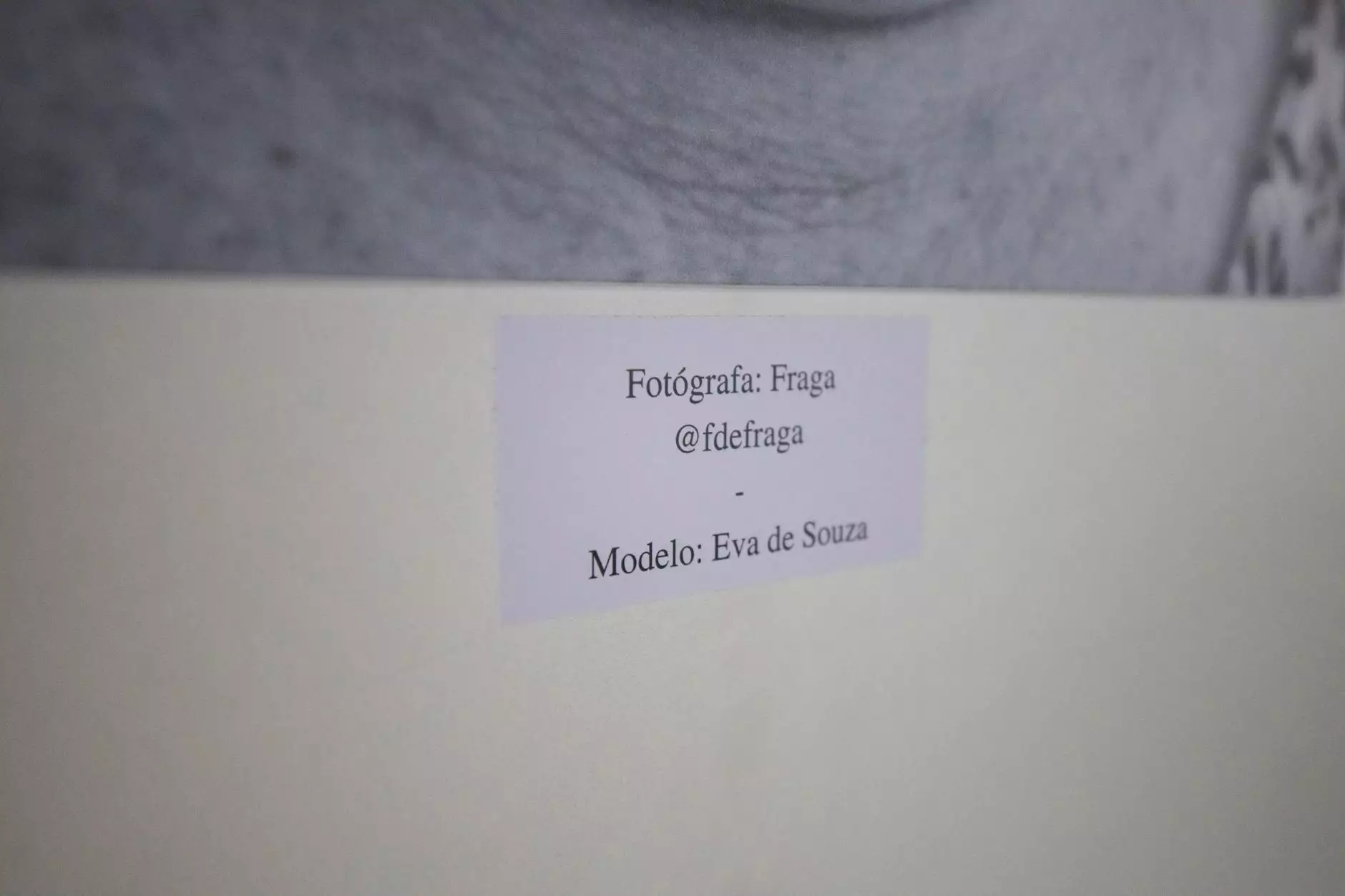
In today's ever-evolving digital landscape, businesses are continuously seeking methods to optimize processes and leverage technology for competitive advantage. One such transformative approach is machine learning data labeling, a critical component of artificial intelligence (AI) development. Understanding this process is essential for companies looking to harness data effectively in order to drive insights and make informed decisions. This article will delve deep into the significance of data annotation, how it operates, and how it contributes to improved business outcomes.
What is Machine Learning Data Labeling?
Machine learning data labeling is the process of annotating data so that machines can understand and learn from it. In simpler terms, it involves tagging or categorizing data—be it images, text, audio, or video—with labels that provide context. This labeled data is then used to train machine learning models, allowing them to recognize patterns, make predictions, and automate decisions.
The Importance of Data Annotation in Machine Learning
Data is the backbone of machine learning algorithms, and the quality of input data directly affects the performance and accuracy of AI models. Here's why data labeling is so vital:
- Boosting Accuracy: Labeled data is essential for supervised learning, where models learn from examples. Proper labeling allows models to identify features accurately, reducing errors.
- Enhancing Model Training: Accurate annotations help train models to generalize better with unseen data, leading to improved predictive capabilities.
- Facilitating Decision-Making: Well-labeled data allows businesses to derive actionable insights, empowering decision-makers with reliable information.
- Increasing Efficiency: High-quality data speeds up the training process of machine learning models, saving time and resources.
Types of Machine Learning Data Labeling
Understanding the various types of data labeling is crucial for businesses looking to implement machine learning solutions. The main types include:
1. Image Annotation
Image annotation involves tagging images with relevant labels. Common practices include:
- Bounding Boxes: Defining regions of interest within an image.
- Semantic Segmentation: Classifying each pixel in an image.
- Keypoint Annotation: Identifying specific points in images relevant for tasks like facial recognition.
2. Text Annotation
Text annotation assigns labels to segments of text. Applications include:
- Sentiment Analysis: Determining the sentiment behind a piece of text, be it positive, negative, or neutral.
- Named Entity Recognition: Identifying proper nouns and classifying them into predefined categories.
3. Video Annotation
Video annotation includes tagging elements within video clips. This is crucial for tasks such as:
- Action Recognition: Identifying activities occurring throughout the video.
- Object Tracking: Following the movements of specific objects across video frames.
4. Audio Annotation
Audio annotation involves tagging audio data for recognition tasks. Techniques include:
- Speech to Text: Converting spoken language into written text.
- Sound Classification: Identifying and labeling different sounds.
The Role of Data Annotation Tools
Using dedicated data annotation tools can significantly streamline the labeling process, making it faster and more efficient. Here are some features that valuable tools offer:
1. User-Friendly Interface
A clean and intuitive interface is vital for users to navigate the tool effortlessly. This enhances productivity and reduces the learning curve.
2. Collaborative Features
Effective tools allow multiple users to collaborate on projects in real-time, ensuring that tasks are completed efficiently and feedback can be integrated promptly.
3. Quality Control Mechanisms
Ensuring high-quality annotations is crucial. Tools equipped with review and quality control features help maintain data accuracy.
4. Integration Capabilities
Data annotation platforms should seamlessly integrate with existing data management systems to facilitate a smooth workflow.
Choosing the Right Data Annotation Platform
When selecting a data annotation platform, businesses need to consider various factors.
1. Types of Data Supported
The platform should support the specific types of data your business utilizes, be it images, text, video, or audio.
2. Scalability
Your chosen platform must be able to grow with your business, accommodating increasing data volumes without compromising performance.
3. Cost-Effectiveness
Analyze the pricing structure of different platforms to ensure it fits your budget while providing the necessary features.
4. Customer Support
Robust customer support is crucial for addressing any queries or issues that may arise during the annotation process.
Implementing Machine Learning Data Labeling in Your Business
To leverage machine learning data labeling effectively, businesses must follow a structured approach:
1. Define Your Objectives
What do you intend to achieve with machine learning? Having clear goals will guide the labeling process and ensure relevance.
2. Assemble High-Quality Data
Start with a clean, diverse dataset that accurately represents the problem you are aiming to solve.
3. Engage Expert Annotators
Utilize skilled annotators who understand the context of your project. This makes a substantial difference in the quality of labeled data.
4. Continuous Monitoring and Iteration
Machine learning is iterative. Continuously assess the quality of your labeled data and refine your approach as needed.
Challenges in Data Labeling and How to Overcome Them
The data labeling process can present several challenges. Recognizing these challenges and developing approaches to overcome them is crucial for successful implementation.
1. Labeling Accuracy
Challenge: Ensuring that data is labeled accurately, as poor quality leads to ineffective models. Solution: Implement quality control checks and use multiple annotators to cross-verify labeled data.
2. Time Consumption
Challenge: Labeling large datasets can be time-consuming and tedious. Solution: Use automation tools and efficient workflows to enhance productivity.
3. Cost Constraints
Challenge: Finding a balance between quality and cost can be difficult. Solution: Choose cost-effective platforms that offer the right blend of features without breaking your budget.
Future Trends in Machine Learning Data Labeling
The field of machine learning is rapidly advancing, and so is the practice of data labeling. Here are some emerging trends to watch for:
1. Automation and AI-Assisted Annotation
As AI technologies evolve, so too will the tools for data labeling. Automation will streamline processes and minimize human error.
2. Crowdsourcing for Enhanced Diversity
Crowdsourcing platforms will continue to grow, allowing businesses to tap into a diverse pool of annotators, enhancing the richness of labeled data.
3. Real-Time Annotation
Demand for real-time annotations will rise as businesses look for rapid results from their data labeling efforts. Innovations in technology will facilitate this shift.
4. Focus on Unlabeled Data
With the vast amounts of unlabeled data available, businesses will invest in semi-supervised and unsupervised learning techniques to derive insights without extensive labeling efforts.
Conclusion
In a world driven by data, machine learning data labeling stands out as a crucial element. As businesses continue to embrace AI, understanding and implementing an effective labeling strategy will determine their success. By leveraging advanced data annotation tools and platforms like those provided by Keylabs.ai, companies can enhance the quality of their datasets, ultimately leading to better machine learning models and more insightful business strategies. Investing in data labeling is no longer optional—it's essential for any business aiming to thrive in the AI era.
Ultimately, the question is not whether to adopt data labeling practices but rather how effectively a business can implement them to stay competitive and innovative.